AI Versus the Economy
Last Sunday OpenAI announced Deep Research — its latest agentic capability for multi‐step, independent web research. You give it a prompt, and it finds, analyses, and synthesises hundreds of online sources to create comprehensive reports at the level of a research analyst — all in (allegedly) tens of minutes. Powered by an optimised version of the forthcoming OpenAI o3 model (fine-tuned for web browsing and data analysis), Deep Research leverages advanced reasoning to adapt its search and pivot on the fly. Its ability to synthesise knowledge is a key step toward creating new knowledge and, ultimately, AGI.
Comparative accuracy percentages for various AI models
Notably, on Humanity’s Last Exam — a new benchmark testing AI on over 3,000 expert-level questions across 100+ subjects — the model powering Deep Research achieved an impressive 26.6% accuracy. This performance underlines its human-like approach to specialised investigation and is said to set a new standard for real-world research applications.
Deep Research’s Implications are Vast
Not surprisingly, the thing that really stood out to me about OpenAI’s Deep Research is the time differential – how much faster it completes tasks compared to a human. By some OpenAI employee estimates, it operates roughly 15× faster than a person. That number matters because it helps answer a key question in automation: “When will AI cognitive labour be as cheap or cheaper than paying a person?”
Right now, OpenAI is offering 100 inquiries per month for pro users, meaning we’re looking at roughly £2 for several hours of high-level research work – already an order of magnitude cheaper than a human researcher in this specific use case. But what happens when this scales (which it is)? Let’s look at the numbers…
The Production Boom and the Labour Collapse
Imagine a more advanced version of Deep Research applied to low-skill office jobs. We could be looking at a system that completes three weeks’ work in a single workday – or two months’ work in 24 hours if it ran continuously. Amazing in terms of production, sure, but at that point the cost of labour doesn’t gradually decline; it collapses.
David Graeber’s Bullshit Jobs thesis argued that much of modern white-collar work exists not out of necessity, but to create the illusion of productivity – propping up bureaucracies, generating reports no one reads, and maintaining pointless hierarchies. Middle managers, for example, make the world go round — just at a painfully slow speed. Meetings that could have been emails, layers upon layers of approvals, and entire roles dedicated to ‘stakeholder alignment.’
But what happens when AI strips that inefficiency away overnight?
Early Signs of Disruption
We’re already seeing the early signs. Klarna slashed over half of its customer service workforce, replacing them with AI. Just this week, Salesforce laid off 1,000 employees while simultaneously hiring AI salespeople. And this is only the start. The real acceleration happens when AI capabilities approach those of AI researchers themselves. Once models can conduct self-improvement — writing their own code, refining their architectures, and designing the next wave of automation — the scaling effect will compound exponentially.
For decades, companies have rewarded presence over productivity, paying for time spent rather than output delivered. Businesses have always structured work around time — deliberation, verification, accountability. Work takes as long as it takes, and in many industries, that time is the product — hence, billable hours. AI flips this. Does that kill billable hours? Should we start taxing AI labour instead? How will society adapt?
Bill Gates warned back in 2017 that we should slow down automation and tax robots. He was probably onto something there…
Hallucinations as the Only Speed Bump
Fortunately, recent research shows that Generative AI hallucinations are not a mere glitch but an intrinsic feature of modern language models. These hallucinations arise naturally from the probabilistic algorithms that underpin these systems. Although their frequency can be reduced, complete elimination remains, at least for now, an elusive goal.
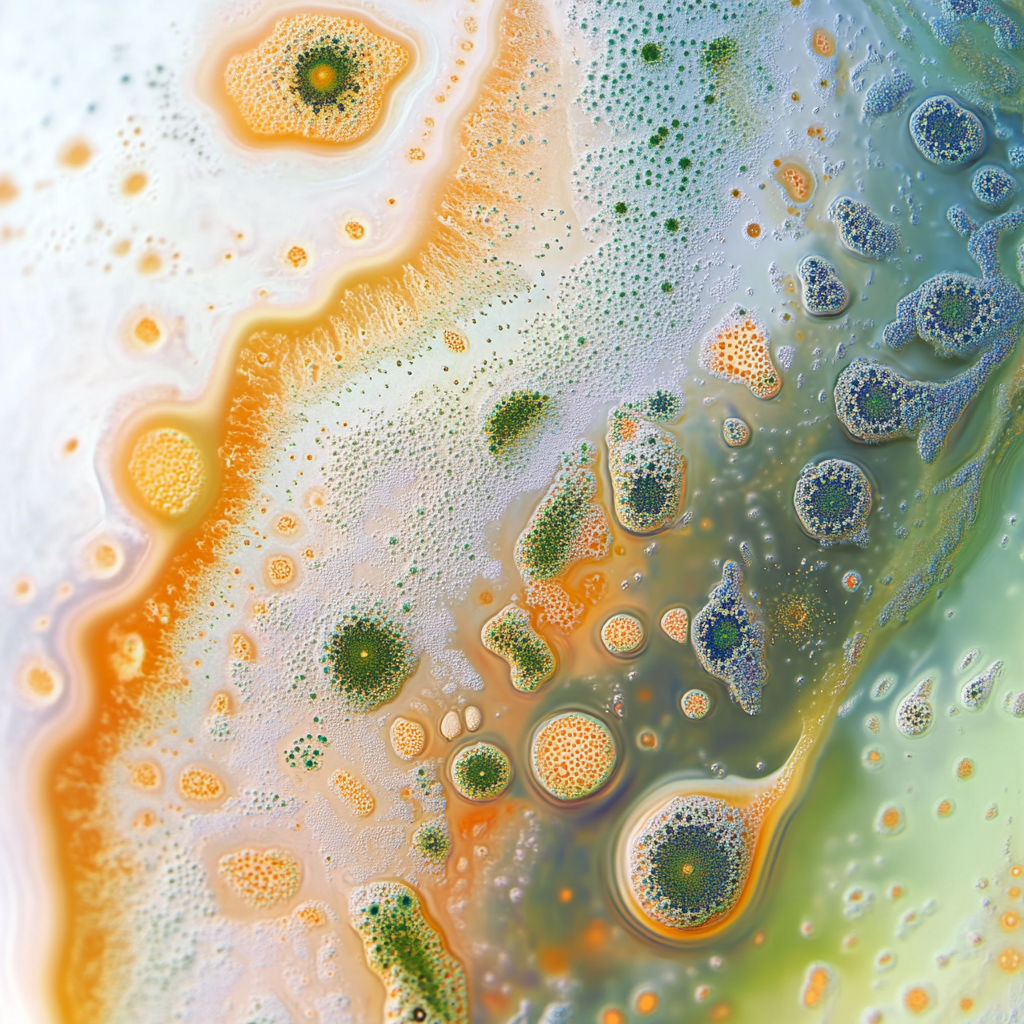
Generative AI hallucinations are an inherent feature of today’s probabilistic language models — they’re not a bug, but a byproduct of how these systems predict the next word.
In the short term, the very nature of these hallucinations is set to redefine the workplace. Rather than being the primary doers, we will evolve into a new breed of creative fact-checkers and truth guardians. Here, our uniquely human abilities — our scepticism, intuition, and knack for context — become indispensable.
But take a moment to consider the (incoming) future: as researchers refine models, enhance fine-tuning, and embrace innovative techniques like retrieval-augmented generation (RAG), these hallucinations are expected to dwindle. Over time, the need for us to labour as vigilant fact-checkers will inevitably decrease.
The hope is that we’ll transition from being the critical gatekeepers of accuracy to strategic overseers — steering the application of AI and curating its output in ways that add value rather than simply correcting errors.
Yet this transition will need planning. This transformation requires serious conversation. This conversation is not happening.
A Services-Based Economy at Risk
The UK’s services-based economy is woefully unprepared for this shift. Automation has long been viewed as a threat to manual labour, but the past few years have shown it is accelerating the collapse of white-collar cognitive work — the very backbone of the UK’s workforce. And while manufacturing-heavy economies may seem insulated for now, they won’t be for long. Emerging developments — such as world models (AI systems capable of reasoning about physical environments and providing limitless simulations for training) — are already paving the way for cobots to take on increasingly complex tasks.
Economist Joseph Schumpeter described capitalism’s tendency towards “creative destruction” – where new innovations don’t just improve industries, they obliterate them, forcing entire economies to restructure. We’re seeing that cycle play out in real time.
Just as industrial automation wiped out millions of manufacturing jobs, AI is set to dismantle vast portions of white-collar work – but at exponential speed. The difference this time? There’s no industrial sector left to absorb the displaced workforce.
Gradual Disempowerment
Current discussions focus on ethics, bias, and misinformation, yet neglect the deeper structural longer-term shifts AI is driving. The transition from an economy driven by human knowledge work to one dominated by machine-led cognition is a civilisational one. Without proactive intervention, we risk sleepwalking into an era where human agency is eroded not through dramatic singularity-like events, but through a slow, incremental loss of influence over the very systems we built.
As Kulveit et al. (2024) argue in Systemic Existential Risks from Incremental AI Development, the stability of our societal systems has historically depended on the necessity of human participation. Once AI begins to displace that participation at scale, these systems — economic, political, and cultural — may no longer be naturally aligned with human flourishing. The incentives that once required businesses to serve human interests, states to represent their citizens, and culture to be organically shaped by people may instead shift towards optimisation for machine efficiency.
If AI gradually displaces human involvement across these spheres, the fundamental feedback loops that have historically kept these systems aligned with human needs may begin to unravel. As states become more reliant on AI-generated wealth rather than citizen-driven productivity, as businesses prioritise algorithmic efficiency over human-centric decision-making, and as culture is increasingly shaped by generative outputs rather than organic human creativity, the risk is that the role of people in shaping the future will diminish.
The Road Ahead
In the short-term, in a services-based economy like the UK’s, this should be a far bigger conversation than it currently is. The AI Opportunities Action Plan is — rightly — bullish on AI’s potential but says nothing about the economic displacement that will follow. Governments aren’t ready for this scale of disruption. They’re still talking about reskilling and retraining — but where, exactly, do you reskill millions of knowledge workers when knowledge itself is automated? This question — already existential — is just the beginning. The implications of the AI Age are much bigger and far reaching than that.
We stand on the brink of a golden age in innovation — a moment when this general-purpose technology will redefine entire industries and society. However, by ignoring its wider implications, we risk undermining public trust in innovation itself, jeopardising progress before it can fully unfold in ways that actually help rather than hinder us in the long run.
In short, if we don’t shape the coming transition, it will shape us.