Act II of the AI Age
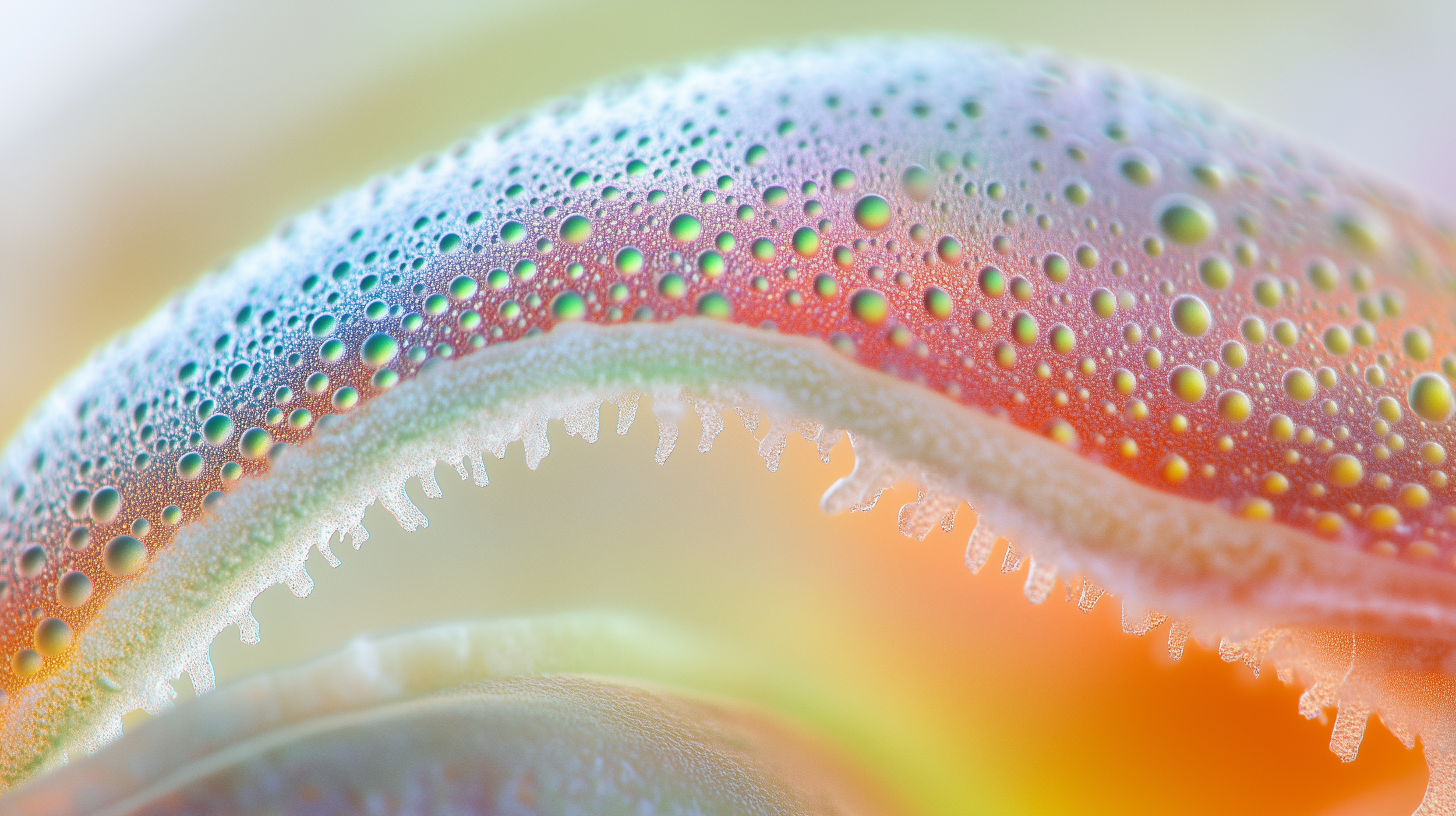
History moves in cycles. Nikolai Kondratiev identified long ago waves of technological and social change, each with an upswing of rapid innovation followed by a second phase of consolidation and integration. As we enter 2025, the AI Age mirrors this pattern. The first act — defined by rapid scaling, shattered benchmarks, and exponential growth — is coming to an end. Now begins Act II: refinement, practical application, and the unhobbling of AI’s potential.
The past two years have seen AI capabilities skyrocket. Timelines collapsed from decades to years. Benchmarks shattered.
However, a general sense of malaise has begun to set in on the wider conversation. OpenAI’s Orion, while highly anticipated, is unlikely to deliver the leap forward many were hoping for. Similarly, expectations were somewhat dampened as OpenAI stated it wants to drop AGI from its clause with Microsoft in order to garner more investment (although Sam Altman insists they still know how to get there).
Regardless of wider developments, in 2025, the real breakthroughs won’t come from bigger models — they’ll come from smarter ways to use them. Increasingly, AI’s potential is being ‘unhobbled’ — a term first coined by Leopold Aschenbrewer in his paper Situational Awareness last year. Unhobbling means fully leveraging existing model capabilities, rather than relying on sheer computational expansion.
From chain-of-thought prompting to modality fusion, to self evolving models and the wider adoption of Retrieval Augmented Generation (RAG), even the largest players are pivoting to focus on practicality and adaptability over scale. At the application layer, this shift is becoming more visible: away from pushing raw capability limits and toward innovating around design, context integration, and user-specific applications.
At the same time, it’s becoming increasingly clear that not every solution needs the world’s knowledge on tap — and in 2025, Smaller Language Models (SLMs) will finally get their moment in the spotlight as businesses seek faster, cheaper, and more efficient AI.
In short, as open-source and proprietary models converge, 2025 will be the year enterprises harness what’s already within reach.
The Great Unhobbling
The models are there for the taking, it's now about making them to work for you.
With existing models no longer improving at an exponential rate, and with the next stage of the game being more about how to leverage LLMs as software rather than on how to make the models themselves better, we’re going to need to begin to measure AI differently.
Multiple-choice tests like MMLU and HellaSwag might have been useful when AI was breaking into new territory, but they’re no longer enough. Models now hit close to 90%+ on these tests, leaving us guessing whether they’re ready for the real world or just optimised to pass exams.
In short, we’ve outgrown traditional benchmarks.
So, what’s next? Companies are turning to new, more complex benchmarks. OpenAI’s ‘SWE-bench Verified’ tasks AI agents with solving real-world coding problems from GitHub — providing reasoning, not just answers. The complexity of these new benchmarks will requires creating comprehensive sandbox environments — see world models below — for the AI to interact with, rather than relying on simple prompts. These are the kinds of measures we need to truly understand AI’s capabilities going forward.
In 2025, we will need to be measuring reasoning, planning, and navigating complexity, not just accuracy. We’re moving from testing what AI knows to testing how AI thinks.
Were the old benchmarks a distraction? Maybe. Some have been criticised as nothing short of paid advertisement. In 2025, it’s time to follow the new lines.
Benchmarks Undone
“We are now starting to saturate our ability to measure some of these systems… it is becoming more and more difficult to evaluate.”
While in the enterprise the unhobbling of models is where we will see the most growth, geopolitically, raw power and compute remain paramount.
Compute power is the foundation of AI progress. Beyond models and algorithms, access to high-performance compute determines who can train, scale, and deploy advanced systems effectively. This creates not just a technological edge but also an economic and geopolitical one.
Nvidia dominates the AI hardware landscape, with 80% of the semiconductor market and its CUDA platform ensuring its GPUs remain central to AI development. Yet this dominance also highlights a growing compute divide: the U.S. controls over 40% of global compute capacity, while Europe lags at less than 10%.
Meanwhile, 75% of infrastructure remains in private hands, creating barriers for smaller players and public institutions. The cost of access is another constraint, with compute costs rising 400% in the last five years.
At the same time, the scale of compute demand is accelerating, with trillion-parameter models expected to define the next era of AI. These systems will require not just larger data centres but entire industrial-scale infrastructures, with training clusters — massive networks of interconnected GPUs and TPUs used to train AI models — projected to exceed $100 billion by 2028 and potentially surpass $1 trillion per cluster by 2030.
As demand for compute grows, disparities in access are becoming increasingly evident. While the U.K. often boasts being one of the top dogs in the AI arena, such a lead will be short lived if it fails to invest more in compute. Hopefully, wise heads will prevail — but lest we forget, this is the U.K., where data centres of “national importance” get blocked for spoiling views of motorways, so a little cynicism is warranted…
That said, there will always be deviations from the rule. Towards the end of last year rumours from China suggested that the nation was finding new, better, cheaper and more innovative ways to train their models in the wake of sanctions which prevented them getting the GPUs needed. Allegedly, many of these models are now as good as their Western counterparts. More evidence that 2025 will be the year of refinement?
Compute Power
Global compute capacity is rapidly expanding, with over 5,500 MW announced in 2024
In late 2024, a wave of announcements about world models — systems that simulate interactive, dynamic virtual environments — highlighted their potential as a defining trend for 2025. At the forefront is Genie 2 by DeepMind, a foundational model capable of generating richly detailed 3D environments from a single image prompt, unlocking new possibilities for training AI agents.
These models solve a fundamental AI challenge: the scarcity of diverse, high-quality training data. By generating limitless virtual environments, world models can create rich synthetic data at scale. They allow AI agents to explore multiple outcomes from the same scenario, ensure consistency over time, and simulate complex physics, object behaviour, and character interactions with remarkable accuracy. This expands training beyond real-world limitations, accelerating learning, improving generalisation, and enabling AI to perform in scenarios where real data is scarce or impractical to collect.
The much-maligned data wall — the growing challenge of finding fresh, high-quality data to train AI models — may have finally met its match. World models, capable of generating synthetic data and training agents in realistic scenarios, ad infinitum, unlock new possibilities for autonomous vehicles, next-generation gaming, and robotics.
With these advancements, 2025 could be the year cobots — collaborative robots — take centre stage, driven by the rise of world models and the expanding capabilities of multimodal AI.
However, fears of model collapse — when the AI snake begins eating itself — will likely become more pronounced as a result of this synthetic data bonanza. But how warranted will they be?
World Models End the Data Wall
What is model collapse?
AI Slop Kills the Internet
In 2025, we will witness a form of the Dead Internet Theory come to life. Deepfakes and AI-generated ‘slop’ — low-effort, synthetic content designed to game engagement algorithms — will create a cycle of diminishing trust and authenticity online, detonating the internet’s utility as a source of information as a result.
As AI models generate more ‘slop’ while being simultaneously trained upon it, the quality and reliability of information online will degrade, fuelling a feedback loop that erodes the internet’s foundation. Users, overwhelmed by synthetic noise and underwhelmed by declining quality, will struggle to distinguish between genuine content and fabrication. As trust deteriorates, skepticism will deepen, driving people to seek information elsewhere.
While initiatives such as The Coalition for Content Provenance and Authenticity (C2PA) are developing countermeasures such as watermarking, these approaches merely scratch the surface of a deeper issue: public trust in technology. Already at a low point, these commendable measures alone won’t rebuild trust in tech.
Unfortunately there are no quick fixes. Transparency, public education, and open dialogue about how AI is being used are desperately needed. If not, the internet, already chock-full of spam and sponsored ads, soon won’t be worth the pixels it's displayed on.
New approaches to information — such as licensing frameworks and AI-driven search tools like Perplexity — will increasingly become the norm. As traditional search engines struggle to surface quality content, publishers will increasingly pivot to these platforms to ensure their work reaches audiences who are bypassing conventional search in favour of AI-curated results.
However, it’s unclear whether this shift will benefit publishing or just be another turn in its death spiral. My bet is on the latter. This marriage of necessity is more likely to accelerate media fragmentation, generate meagre revenue (OpenAI’s deal with Dotdash Meredith is equal to about 1% of the publisher’s total revenue), and remain plagued by issues of transparency and concentrated power.
All of above, blended within a bot-dominated distortion of what Tim Berners-Lee envisioned as the Internet 4.0, as autonomous agents begin to populate a World Wide Web where no-one can really know what is and is not ‘real’.
The internet as we know it is dying. In 2025, both creators and consumers will have to navigate a world where authenticity is a commodity, and trust becomes harder to earn. Blockchain anyone?
Nearly 2x people more likely to say innovation is poorly managed vs well managed.
News of Meta and Palantir joining forces to bring the Llama AI model to U.S. government and defence operations reflects a broader shift toward platformisation in the public sector. As government institutions increasingly outsource AI capabilities to private technology platforms, we are witnessing a transformation in how public services operate.
The reasons why are clear. Estimates suggest that AI’s cumulative GDP impact in public sectors such as health and education is projected to reach 6% over several decades, driven by enhanced productivity and reduced operational costs.
Meanwhile, other estimates say that AI could boost educational attainment levels by around 6 per cent through a combination of improving average attainment of individual students and by enabling more students to progress to higher levels of education. This effect has been found to be even more predominant among lower-performing students, making education more equitable to boot.
Similarly, AI-driven job-matching services are being implemented in countries like France, where public platforms predict hiring demand, showcasing a direct benefit of platformisation in improving labour-market efficiency.
Needless to say, these examples only scratch the surface of what AI can bring to the public sector.
Yet, as governments deepen their reliance on private AI platforms, questions around data privacy, governance, and dependency loom large. Will this trend enhance public services, or will it hand too much control to private entities shaping critical infrastructure?
Just like publishing, the public sector has little choice in the matter. However, unlike publishing the cost-benefit equation for governments is far more favourable. AI’s ability to improve efficiency, reduce costs, and modernise legacy institutions makes this a shift that is clearly weighted in its favour (for now).
In short, 2025 will be defined by public-private collaboration, with the public sector leveraging AI-driven platforms to meet the demands of a modern, AI-first society.
Platformisation Takes Hold
“How you understand, master and harness this technology revolution will define the place of this country and the shape of the world.”
While the word agentic might make language purists wince at its mulish mix of Latin and Greek roots, it’s a word we’ll be hearing a lot more in 2025 as AI agents transition from concept to reality. This year, these artificial workers will step into the workforce, moving beyond isolated, task-specific roles to become part of networked systems that collaborate, share intelligence, and operate at scale.
This shift marks the rise of the agentic enterprise, where Multi-Agent Systems (MAS) allow AI to coordinate dynamically — sometimes autonomously, sometimes with a human-in-the-loop depending on the task) — while working together to achieve broader objectives.
Frameworks like OpenAI’s Swarm and Langraph by Langchain are key catalysts for this shift. Unlike traditional AI models operating in isolation, these frameworks allow for multiple AI agents to work in concerto, coordinating tasks dynamically.
Insight retrieval, content creation, employee care, the list goes on. AI capabilities are converging, seamlessly transforming reports into presentations, generating job advertisements the moment a new hire is needed, and handling customer inquiries with increasing autonomy.
Meanwhile, on the other side of the aisle, AI agents will start making purchases on behalf of consumers — potentially ushering in a new era where brands must market not just to humans, but to machines (when the agent’s primary directive is to buy a Pepsi how will you convince it to buy a Coke?).
In any case, for AI agents to be effective, they need to balance simplicity, adaptability, and autonomy. The best implementations avoid unnecessary complexity, and are built on augmented LLMs with retrieval, tools, and memory — ensuring they can access knowledge and take action efficiently.
While fully autonomous systems are unlikely to see widespread deployment until late 2025, in the meantime, we’ll see more semi-autonomous agents — operating with a human-in-the-loop when needed. At the same time, tools like Claude’s ‘Computer Use’ feature — which allows AI to take control of a user’s desktop — will help normalise agentic interactions for the wider population.
The agents are here. The only question in 2025 is what tasks you choose to trust them with.
Planet Agent
In your opinion, which tasks are agents best suited to perform today?
The end-of-year arrival of OpenAI’s Sora and Google’s Veo 2 reminded us that one of the most pertinent trends in Generative AI has been its ability to lower the entry bar to creativity. This is set to become even more true in 2025.
Far from being the death of creative, we have witnessed a Cambrian explosion in images, video, songs, and much else since the arrival of Generative AI tools like Midjourney. With video and world models now entering the conversation, this trend will be compounded further still.
As such, we will begin to see the arrival of content creation at the individual scale, where people will be able to create and customise their own entertainment — be it music, video or even gaming — at unprecedented levels.
At the same time, audiences will be able to consume in the format that suits them best. In 2025, we will see content will become increasingly fluid, adapting in real-time to fit the preferences, formats, and contexts that suit each user best.
Such developments will inevitably intersect with other sectors such as education. As seen by NotebookLM last year, the ability to generate content at a personal level will unlock new ways of learning. Similarly, the intersection of world models, GenAI physics engines and new XR hardware will take us over — dare I say it — the metaverse event horizon.
Elsewhere, industries protecting such content from web scraping will become more pronounced as creators become more aware of the fact that their content is at risk of being fed to The Machine.
All the while, these new AI creative tools will become even more intuitive to use, further unleashing creativity and democratising it anyone and everyone with a good idea.
The result? Unbridled, unhobbled, creative unbounded.
Entertainment Democratised
“Stop thinking of AI as doing the work of 50% of the people. Start thinking of AI as doing 50% of the work for 100% of the people.”